It sounds like science fiction, but data analysts are using the tools of their trade to predict some types of crime—information that could be used to keep people safe.
Tong Wang, assistant professor of management sciences in the University of Iowa’s Tippie College of Business, is on the cutting edge of developing algorithms to predict a variety of human behavior. Her goal, she says, is to make the mass of numbers and formulae that she studies relevant to the lives of everyday people.
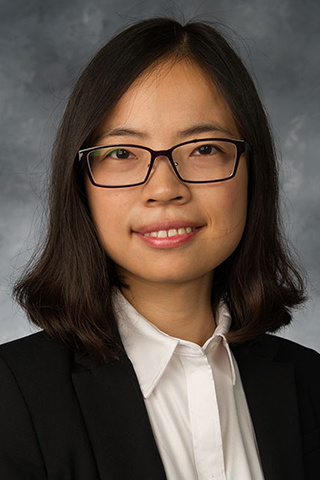
“I want to develop algorithms that are easy for people who aren’t trained in algorithms to understand,” she says. “I don’t want people to see analytics as a black box. People don’t understand black boxes, and so they’re reluctant to adopt them. Transparency of a model is critical.”
One of Wang’s specialties is using analytics to improve public safety by predicting criminal behavior patterns. As a graduate student at the Massachusetts Institute of Technology, she worked on a project with the Cambridge, Massachusetts, police department that was designed, in part, to replace the wisdom of a longtime officer who was about to retire. Rich Sevieri was nearing the end of his 30-year career, and his younger colleagues knew they’d sorely miss his experience and expertise in spotting patterns of criminal behavior.
“He had seen so much, he could tell just by how a person was standing if they were carrying a gun,” says Wang.
Sevieri’s fellow officers knew also that researchers had been using data analytics to identify behavioral patterns that otherwise would be difficult to track. They approached Wang and other researchers at MIT to see if an algorithm could be created to duplicate Sevieri’s wisdom. Wang was part of the team that developed an algorithm to identify crime series—or patterns of behavior by the same individual or group during home break-ins.
Wang and her MIT colleague Cynthia Rudin asked Sevieri questions about the factors he discovered were most important in determining a crime pattern—such as the neighborhood, time of day the break-in occurred, method of entry, and the weather. Did the criminals focus on homes or apartments? Did they steal one type of particular item? Did they ransack the house or leave things where they were?
Detecting these series can be an important step in predictive policing, Wang says, because uncovering a pattern helps law enforcement locate and stop the offenders.
Eventually, Wang and Rudin built an algorithm that combines general characteristics across different crime series and distinctive characteristics for each series. Their method was used on more than 7,000 historical crimes that happened within a 10-year period. The researchers characterized the criminals’ mode of operation (MO) in each break-in, looking for similarities that the algorithm could connect.
In the past, this kind of search was done by hand, with detectives poring over databases, notes, and case files looking for similarities. The work took days or weeks, and sometimes the police never found the connections. But the algorithm needs only seconds to analyze the data and spot the similarities.
Wang says other algorithms have been developed to find patterns, but take into account only a limited number of factors and can predict crimes based only on location and time factors in the past. The algorithm that Wang and her research team built incorporates so many more factors—including the MO—and is much more flexible and precise in its predictive capability.
Once the algorithm identifies a pattern, police can use other resources to help solve the crime. For instance, detectives can use security camera footage from businesses in the area to see if the same people are present at the location of separate crimes.
To test the algorithm, Wang and her research team used data the Cambridge Police Department had gathered from investigations between 1997 and 2006 and in which detectives spotted 51 crime series. The algorithm corrected some misidentified crimes and found other crime series in the data that detectives had missed during earlier investigations.
“There was a pattern of break-ins in west Cambridge and east Cambridge that the algorithm saw right away,” Wang says. “When Rich (Sevieri) saw that, he came over and shook our hands. He said it took months for him to spot that pattern. He was supportive of our work right from the start, but that really won him over.”
The work with Sevieri was impressive enough that The Boston Globe wrote a feature about it.
Wang has since added Tippie doctoral student Yunyi Li to her team, and their next avenue for research is using analytics to prevent a crime from happening. They will use the Cambridge database to build an algorithm that predicts the next location a serial criminal will hit according to their previous crimes. With that information, public safety officials can take steps to prevent it.
“You need to accurately detect which crimes are committed by the same person in order to study the characteristics and predict future crimes,” Wang says.